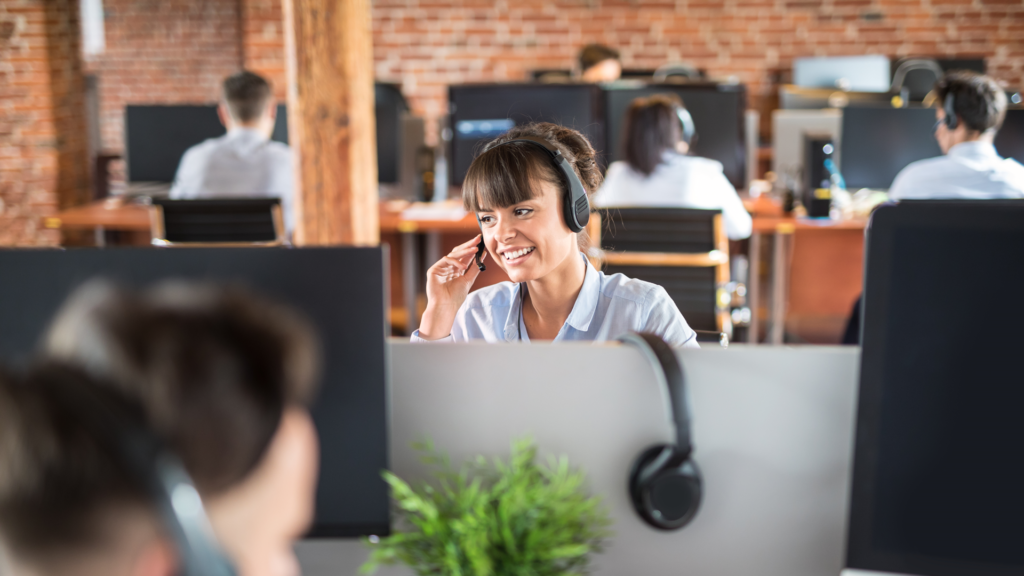
Back Story
I recently judged the L&D category for the CCMA’s national awards. In the process, I noticed quite a few submissions highlighting how they needed to recruit more front-line advisors in response to a significant increase in inbound volumes.
This reconfirmed that human contact remains a growing priority for consumers. Moreover, I don’t see the kind of life challenges driving this need as ones likely to go away anytime soon. In fact, McKinsey stated in their 2024 State of Customer Care research that “57 percent of leaders in global enterprises expect call volumes to increase by as much as one-fifth over the next one or two years.”
So, let’s assume it’s going to be a persistent leadership challenge. One that is already framing the debate about where organisations focus their efforts to leverage the latest generation of AI. To my mind this is to optimise advisor performance and protect their well-being/resilience in the context of managing longer, more intense and demanding conversations.
Those awards entries indicated organisations still instinctively gravitate towards adding headcount whenever inbound volumes grow substantially. This is problematic. Both in terms of the cost of employing more people and their availability as a recruitable resource. Clearly there are constraints to this approach.
Instead, I suggest we need to think about things differently and look more creatively at opportunities to get more from existing capacity. Not by simply expecting front line teams to work harder. But in how they are used more effectively as the result of optimised contact strategy.
What might this look like?
Understanding Why Customer Want Service
Let’s start by defining this mission as protecting the availability of human delivered customer service for interactions that need uniquely human talents. These include, resolving customer needs that are complex, emotive, urgent or require relationship repair/building.
Incidentally, human-delivered interaction is also a great opportunity to surface cross and upsell opportunities: something lost when customers are in self-serve mode. This is a growing trend according to one of my favourite analysts Nicolas de Kouchkovsky who estimates ‘20-25% of contact center positions now play a direct role in generating revenue’.
But back to the mission. Why do we even need to think about ‘protecting availability’? Surely knowing the size of your advisor community is sufficient? Not really. To borrow from the language of workforce planning it’s all about managing shrinkage. What appears to be your capacity is seldom your actual capacity because circumstances and competing demands eat into that availability.
In a similar way, protecting the availability of human delivered customer service requires an intentional strategy to reduce or entirely remove anything that shrinks this capacity.
Workforce planners look at sickness, absenteeism, holidays, training etc as the typical causes of shrinkage. However, the culprits that I’m calling out are different and not as easy to quantify since they are not measured with the same consistency.
Unfortunately, the long-standing practice of just tracking how customer demand fluctuates over time does not tell us when human delivered service is needed. Or more to the point, when it should not be used and is therefore being wasted.
The good news is that the latest generation of NLP (Natural Language Processing) can now tell us so much more about customer demand when deployed with an effective methodology.
Let’s start with how to identify the use cases for human delivered customer service. The analysis starts with an understanding of why customers make contact. This is done by mapping types of customer need and the associated intents within each.
Then within the universe of all inbound demand, those requiring human assistance are identified in two ways. First by applying the lens of complexity, emotion, urgency and relationship goodwill. Which types of customer need and intents involve a mix of these characteristics? Descriptions can be further qualified by applying sentiment and inferred emotion analysis which helps bring the mood of a conversation to life.
Once these are described, AI models can be trained on these datasets and used to track the degree to which human assistance is being optimally used within inbound volumes.
Incidentally, these AI models have many associated use cases. Such as enriching dynamic scheduling, priority routing, agent assist interventions, quality monitoring and personalised coaching. All of which complement the ability to amplify human to human interaction for memorable experiences and outcomes.
Unsurprisingly these types of conversation are also the ones that matter most to customers. Therefore, elevating their resolution to become a prime collective accountability is crucial given the high stakes involved.
In relation to this, Forrester just announced the lowest ever score in its annual CX Index score in the US. While IPSOS reported just 42% of UK customer as being satisfied with their service experiences. This implies making the most of human delivered service is going to matter even more as organisations recommit to doing better. Or face heightened risk of reputational damage and churn.
Being able to describe the use cases for human assisted service clarifies the search criteria to effectively trawl customer interaction recordings and estimate volumes and related patterns of demand. This insight becomes even more crucial if inbound volumes continue to rise as suggested earlier and organisations try to understand what proportion really qualify for their limited human delivered service.
Strategies to Optimise Availability of Human Delivered Customer Service
Almost certainly there won’t be enough headcount to go around because at this point nothing has been done to stop other factors eating away at capacity. These include:
- Using live assistance for inbound conversations that have zero value to either customer or organisation. This is often called failure demand and once identified can be reduced or eliminated through root cause analysis and redesign.
- Using live assistance for customer needs that can be autonomously resolved by customers. Typically, these type of needs and intents are characterised by low/no levels of complexity, emotion, urgency or need for relationship repair. Even so, Gartner analysis shows full resolution within self-service channels remains far too low at just 13%. This can be fixed by investing in human centered design and agile cross functional collaboration.
- Using live assistance for customer needs that could be resolved through proactive communication before adding to inbound contact volumes. This approach unearths predictable moments of need in customer journeys that can be anticipated and proactively resolved using automated voice or text messaging. Again, well trained AI models excel at this type of analysis.
Eliminating failure demand combined with growth in self-service and proactive messaging use cases will free up capacity for human delivered service. Of course, a percentage of demand for human assistance will always emerge from self-service and proactive messaging given customer needs for reassurance, or when a normally simple self-service journey becomes unexpectedly complex and emotive.
These kinds of ‘in the moment’, situational needs are all but impossible to anticipate. They need to be estimated and factored into planning assumptions.
However, what can be affected are instances in which customers are forced to join an inbound queue. As caused by poor design or other factors which compromise the effectiveness of a self-serve or proactive messaging journey. These need to be continuously scanned for and quickly cleaned up to support the mission of optimal availability.
As a final area of opportunity, it is worth exploring where it is possible to reduce any administrative overhead before, during and after a customer conversation. Again, for the purpose of conserving advisor availability.
Typically, solutions to these challenges are enabled through technology such agent-assist which can brief the advisor before a conversation starts by sharing anything learnt about customer needs and intent from an initial triage process and reducing customer irritation at having to repeat themselves. Wrap up can also be streamlined by using GenAI to produce customer communications and call summarisations.
Behavioural modelling is another interesting opportunity for analytics. Which advisors are delivering the best CX outcomes in relation to specific needs and intents? What are they doing right? Can others learn to imitate their techniques? Apart from the benefit of increasing the consistency and quality of customer conversation, time is also typically saved given their expertise.
Call To Action
We are in a period of rising interaction volumes across all channels. McKinsey research shows demand for human assistance is more evenly distributed across the generations than is commonly assumed.
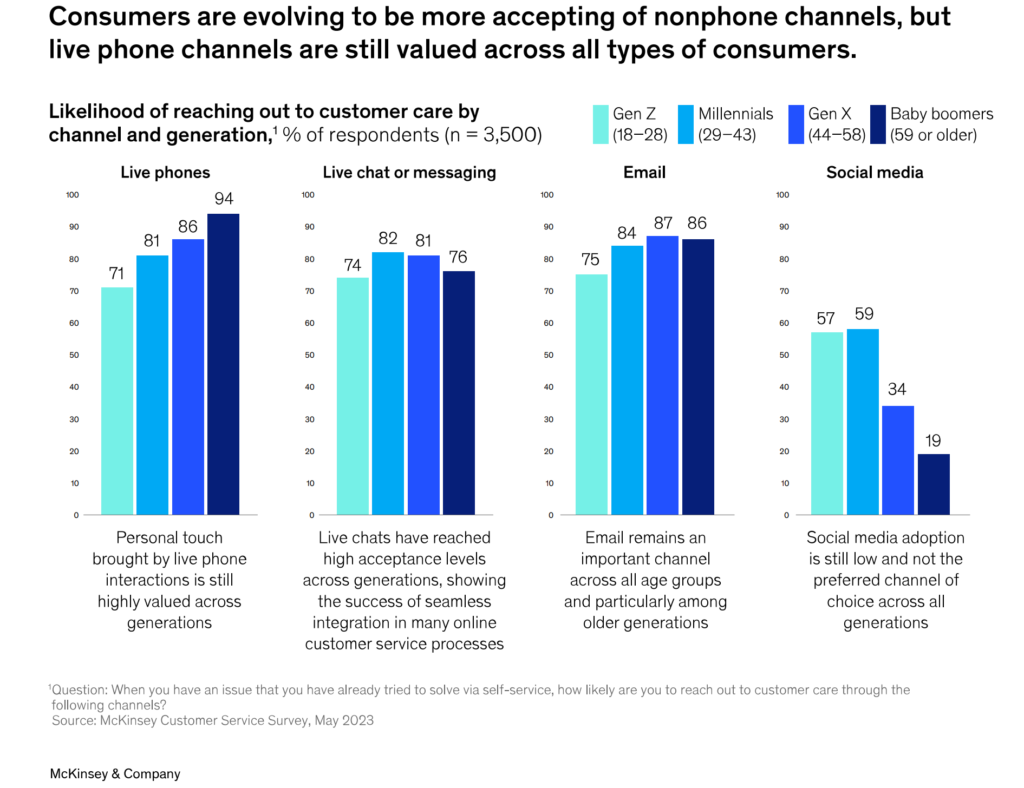
Headcount is expensive hence the need to optimise it by setting a strategic intent of using it for its intended use cases only. An optimised contact strategy requires persistent scanning, interpretation and response to dynamically evolving inbound demand.
This provides the insight required to ensure:
- Failure demand is controlled.
- Self-service is designed to fulfil the potential many believe it is capable of scaling to
- Proactive service becomes the norm rather than the exception.
- Conversational best practice is harvested and rapidly adopted across front line teams
The alternative is already familiar. Long wait times, missed SLAs, poor resolution rates and over-stressed advisors seeking a better work:life balance. In these circumstances, any ROI from customer experience simply fades away.
But that need not be the case with an intentional focus on optimising availability of your human delivered customer service. If you want to learn more about the methodology that drives the approach and how to embed it in your own service organisation, let me know via the form below.
As ever, thanks for your attention and interest.